Appearance Optimization of Environmental Conditioning Equipment: Multi-Physical Field Coupling & Optimization Algorithm Solution
Abstract
For indoor air quality control equipment optimization, data on ACs, humidifiers, and purifiers is collected. Shape optimization models are built for each to analyze shape factor impacts on functions and obtain optimal shapes and sizes[1]. Then a 3-in-1 device appearance optimization model is established to maximize energy efficiency, human comfort, purification, and humidification effects.
First, air conditioning temperature field optimization analyzes unit placement, inlet/outlet, and wind speed/volume. With heat conduction, Navier-Stokes, and energy equations, a model of indoor airflow and temperature is built. Particle swarm optimizer, finite difference discretizes, SIMPLE solves, and adaptive mesh improves accuracy for uniform temperature.
Secondly, for air purifier shape optimization, a model linking purification effect and shape is set up, with a multi-physical coupling model of particle motion, airflow, pollutant diffusion, and filtration efficiency for efficient purification. The finite difference method discretizes relevant equations and turns partial differential equations into algebraic ones for solving, and the gradient descent method[2] is employed to obtain optimal shape parameters, ultimately finding the best shape and size to maximize purification.
Thirdly, for humidifier performance optimization, consider its role in vapor processes. Build a math model integrating evaporation rate, diffusion, and shape geometry for multi-quantity coupling. Use simulated annealing[3] to iteratively find the optimal, achieving precise humidification control and optimal shape/size.
Finally, integrate models of the previous three problems for the three-in-one environmental regulator. Build a hierarchical optimization model with multi-physical field coupling[4]. Use multi-objective particle swarm genetic algorithm for overall performance optimization[5]. Explore field-volume coupling-based hierarchical nested optimization algorithm to handle multifunctional integration.
This study constructs a full optimization design system. Despite physical model simplification limitations, it's improvable. The results are extendable to other environmental conditioning equipment optimization.
References
[2] Wang, J. C., Liu, Y. L., Zhang, P., Liu, M. H., & Zhao, X. H. (2024). Privacy-preserving scheme for SVM training based on small batch stochastic gradient descent method[J]. Information Security Research, 10(10).
[3] Qiu, Y., Zhu, P., Su, Y., Mollal, A., & Ren, Y. (2024). Optimization of NO₂ air monitoring stations based on spatial simulated annealing algorithm: a case study of southwestern Fujian urban agglomeration[J]. Journal of Ecology. (Published online: 2024-11-21 16:20:54).
[4] Zeng, B. (2024). Urban Construction Theory Research (Electronic Edition), 29. https://doi.org/10.19569/j.cnki.cn119313/tu.202429038
[5] Yuan, H., Ke, Y., Xiang, K., Fan, L., Ma, H., Wang, C., & Xilei. (2024). Hierarchical optimal scheduling of wind-gas-fire-storage multi-source systems for peak shifting[J]. Hydroelectric Power Generation. (First published online: 2024-11-01 10:48:24).
[6] Li, X., Gui, Y., Jia, H., Li, X., Hao, M., Zhong, Y., & Zhang, L. (2024). Thermal-fluid coupling simulation model of flat slab slurry grouting in cracks with polymer slurry[J]. Engineering Mechanics. (Web debut: 2024-11-08 14:52:53).
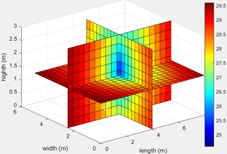

This work is licensed under a Creative Commons Attribution 4.0 International License.
Copyright for this article is retained by the author(s), with first publication rights granted to the journal.
This is an open-access article distributed under the terms and conditions of the Creative Commons Attribution license (http://creativecommons.org/licenses/by/4.0/).