Algorithmic Resistance and Online Privacy: Extending the Meta-UTAUT Model with Particular Privacy Concerns
-
Abstract
This study delved into the perceived benefits and privacy concerns individuals face when interacting with algorithms, and explored their relation to algorithmic resistance. Based on technology acceptance research and online privacy studies, an extended Meta-UTAUT model was proposed. A total of 434 valid samples were obtained in China. The results show that perceived benefits (including performance expectancy, effort expectancy, social influence, and facilitating conditions) are negatively related to algorithmic resistance attitude. Moreover, concerns for technology and financial privacy are positively related to algorithmic resistance intention.This result identifies the aspects of privacy highly esteemed in the interaction between individuals and algorithms. Finally, the contributions, practical and theoretical significance, and limitations of this study were discussed.
References
Akter, S., McCarthy, G., Sajib, S., Michael, K., Dwivedi, Y. K., D’Ambra, J., & Shen, K. N. (2021). Algorithmic bias in data-driven innovation in the age of AI. International Journal of Information Management, 60, 102387. https://doi.org/10.1016/j.ijinfomgt.2021.102387
Alkhowaiter, W. A. (2022). Use and behavioural intention of m-payment in GCC countries: Extending meta-UTAUT with trust and Islamic religiosity. Journal of Innovation & Knowledge, 7(4), 100240. https://doi.org/10.1016/j.jik.2022.100240
Aloudat, A., Michael, K., Chen, X., & Al-Debei, M. M. (2014). Social acceptance of location-based mobile government services for emergency management. Telematics and Informatics, 31(1), 153–171. https://doi.org/10.1016/j.tele.2013.02.002
Aw, E. C.-X., Tan, G. W.-H., Cham, T.-H., Raman, R., & Ooi, K.-B. (2022). Alexa, what’s on my shopping list? Transforming customer experience with digital voice assistants. Technological Forecasting and Social Change, 180, 121711. https://doi.org/10.1016/j.techfore.2022.121711
Awad & Krishnan. (2006). The Personalization Privacy Paradox: An Empirical Evaluation of Information Transparency and the Willingness to Be Profiled Online for Personalization. MIS Quarterly, 30(1), 13. https://doi.org/10.2307/25148715
Ayuning Budi, N. F., Adnan, H. R., Firmansyah, F., Hidayanto, A. N., Kurnia, S., & Purwandari, B. (2021). Why do people want to use location-based application for emergency situations? The extension of UTAUT perspectives. Technology in Society, 65, 101480. https://doi.org/10.1016/j.techsoc.2020.101480
Balakrishnan, J., Abed, S. S., & Jones, P. (2022). The role of meta-UTAUT factors, perceived anthropomorphism, perceived intelligence, and social self-efficacy in chatbot-based services? Technological Forecasting and Social Change, 180, 121692. https://doi.org/10.1016/j.techfore.2022.121692
Bandyopadhyay, S. (2011). Antecedents And Consequences Of Consumers Online Privacy Concerns. Journal of Business & Economics Research (JBER), 7(3). https://doi.org/10.19030/jber.v7i3.2269
Banker, S., & Khetani, S. (2019). Algorithm Overdependence: How the Use of Algorithmic Recommendation Systems Can Increase Risks to Consumer Well-Being. Journal of Public Policy & Marketing, 38(4), 500–515. https://doi.org/10.1177/0743915619858057
Baruh, L., Secinti, E., & Cemalcilar, Z. (2017). Online Privacy Concerns and Privacy Management: A Meta-Analytical Review: Privacy Concerns Meta-Analysis. Journal of Communication, 67(1), 26–53. https://doi.org/10.1111/jcom.12276
Becker, G. S., & Murphy, K. M. (1988). A Theory of Rational Addiction. Journal of Political Economy, 96(4), 675–700. https://doi.org/10.1086/261558
Boyd, D. M., & Ellison, N. B. (2007). Social Network Sites: Definition, History, and Scholarship. Journal of Computer-Mediated Communication, 13(1), 210–230. https://doi.org/10.1111/j.1083-6101.2007.00393.x
Bruns, A. (2019). Filter bubble. Internet Policy Review, 8(4). https://doi.org/10.14763/2019.4.1426
Bucher, T. (2017). The algorithmic imaginary: Exploring the ordinary affects of Facebook algorithms. Information, Communication & Society, 20(1), 30–44. https://doi.org/10.1080/1369118X.2016.1154086
Burrell, J. (2016). How the machine ‘thinks’: Understanding opacity in machine learning algorithms. Big Data & Society, 3(1), 2053951715622512. https://doi.org/10.1177/2053951715622512
Chatterjee, S., Rana, N. P., Khorana, S., Mikalef, P., & Sharma, A. (2023). Assessing Organizational Users’ Intentions and Behavior to AI Integrated CRM Systems: A Meta-UTAUT Approach. Information Systems Frontiers, 25(4), 1299–1313. https://doi.org/10.1007/s10796-021-10181-1
Chen, H.-T. (2018). Revisiting the Privacy Paradox on Social Media With an Extended Privacy Calculus Model: The Effect of Privacy Concerns, Privacy Self-Efficacy, and Social Capital on Privacy Management. American Behavioral Scientist, 62(10), 1392–1412. https://doi.org/10.1177/0002764218792691
Chen, K. (2024). If it is bad, why don’t I quit? Algorithmic recommendation use strategy from folk theories. Global Media and China, 9(3), 344–361. https://doi.org/10.1177/20594364231209354
Chopdar, P. K. (2022). Adoption of Covid-19 contact tracing app by extending UTAUT theory: Perceived disease threat as moderator. Health Policy and Technology, 11(3), 100651. https://doi.org/10.1016/j.hlpt.2022.100651
Christin, A. (2020). The ethnographer and the algorithm: Beyond the black box. Theory and Society, 49(5–6), 897–918. https://doi.org/10.1007/s11186-020-09411-3
Cook, K. S., Cheshire, C., Rice, E. R. W., & Nakagawa, S. (2013). Social Exchange Theory. In J. DeLamater & A. Ward (Eds.), Handbook of Social Psychology (pp. 61–88). Springer Netherlands. https://doi.org/10.1007/978-94-007-6772-0_3
Cotter, K. (2024). Practical knowledge of algorithms: The case of BreadTube. New Media & Society, 26(4), 2131–2150. https://doi.org/10.1177/14614448221081802
Cyberspace Administration of China. (2022). Provisions on the Management of Algorithmic Recommendations in Internet Information Services. Retrieved from https://www.cac.gov.cn/2022-01/04/c_1642894606364259.htm. Accessed November 20, 2024
Davis, F. D. (1989). Perceived Usefulness, Perceived Ease of Use, and User Acceptance of Information Technology. MIS Quarterly, 13(3), 319. https://doi.org/10.2307/249008
Davis, F. D., Bagozzi, R. P., & Warshaw, P. R. (1992). Extrinsic and Intrinsic Motivation to Use Computers in the Workplace1. Journal of Applied Social Psychology, 22(14), 1111–1132. https://doi.org/10.1111/j.1559-1816.1992.tb00945.x
DeVito, M. A., Gergle, D., & Birnholtz, J. (2017). “Algorithms ruin everything”: #RIPTwitter, Folk Theories, and Resistance to Algorithmic Change in Social Media. Proceedings of the 2017 CHI Conference on Human Factors in Computing Systems, 3163–3174. https://doi.org/10.1145/3025453.3025659
Dhagarra, D., Goswami, M., & Kumar, G. (2020). Impact of Trust and Privacy Concerns on Technology Acceptance in Healthcare: An Indian Perspective. International Journal of Medical Informatics, 141, 104164. https://doi.org/10.1016/j.ijmedinf.2020.104164
Diamantopoulos, A., & Siguaw, J. A. (2006). Formative Versus Reflective Indicators in Organizational Measure Development: A Comparison and Empirical Illustration. British Journal of Management, 17(4), 263–282. https://doi.org/10.1111/j.1467-8551.2006.00500.x
Dienlin, T., Masur, P. K., & Trepte, S. (2023). A longitudinal analysis of the privacy paradox. New Media & Society, 25(5), 1043–1064. https://doi.org/10.1177/14614448211016316
Dinev, T., & Hart, P. (2006). An Extended Privacy Calculus Model for E-Commerce Transactions. Information Systems Research, 17(1), 61–80. https://doi.org/10.1287/isre.1060.0080
Donmez-Turan, A. (2019). Does unified theory of acceptance and use of technology (UTAUT) reduce resistance and anxiety of individuals towards a new system? Kybernetes, 49(5), 1381–1405. https://doi.org/10.1108/K-08-2018-0450
Duan, S. X., & Deng, H. (2022). Exploring privacy paradox in contact tracing apps adoption. Internet Research, 32(5), 1725–1750. https://doi.org/10.1108/INTR-03-2021-0160
Durnell, E., Okabe-Miyamoto, K., Howell, R. T., & Zizi, M. (2020). Online Privacy Breaches, Offline Consequences: Construction and Validation of the Concerns with the Protection of Informational Privacy Scale. International Journal of Human–Computer Interaction, 36(19), 1834–1848. https://doi.org/10.1080/10447318.2020.1794626
Dwivedi, Y. K., Hughes, L., Ismagilova, E., Aarts, G., Coombs, C., Crick, T., Duan, Y., Dwivedi, R., Edwards, J., Eirug, A., Galanos, V., Ilavarasan, P. V., Janssen, M., Jones, P., Kar, A. K., Kizgin, H., Kronemann, B., Lal, B., Lucini, B., … Williams, M. D. (2021). Artificial Intelligence (AI): Multidisciplinary perspectives on emerging challenges, opportunities, and agenda for research, practice and policy. International Journal of Information Management, 57, 101994. https://doi.org/10.1016/j.ijinfomgt.2019.08.002
Dwivedi, Y. K., Rana, N. P., Jeyaraj, A., Clement, M., & Williams, M. D. (2019). Re-examining the Unified Theory of Acceptance and Use of Technology (UTAUT): Towards a Revised Theoretical Model. Information Systems Frontiers, 21(3), 719–734. https://doi.org/10.1007/s10796-017-9774-y
Dwivedi, Y. K., Rana, N. P., Tamilmani, K., & Raman, R. (2020). A meta-analysis based modified unified theory of acceptance and use of technology (meta-UTAUT): A review of emerging literature. Current Opinion in Psychology, 36, 13–18. https://doi.org/10.1016/j.copsyc.2020.03.008
Ettlinger, N. (2018). Algorithmic affordances for productive resistance. Big Data & Society, 5(1), 2053951718771399. https://doi.org/10.1177/2053951718771399
Fan, J., Shao, M., Li, Y., & Huang, X. (2018). Understanding users’ attitude toward mobile payment use: A comparative study between China and the USA. Industrial Management & Data Systems, 118(3), 524–540. https://doi.org/10.1108/IMDS-06-2017-0268
Fast, N. J., & Jago, A. S. (2020). Privacy matters… or does It? Algorithms, rationalization, and the erosion of concern for privacy. Current Opinion in Psychology, 31, 44–48. https://doi.org/10.1016/j.copsyc.2019.07.011
Fishbein, M., & Ajzen, I. (1975), Belief, Attitude, Intention and Behavior: An Introduction to Theory and Research, Addison-Westley, Reading, MA. Rereieved from https://www.jstor.org/stable/40237022
Fogel, J., & Nehmad, E. (2009). Internet social network communities: Risk taking, trust, and privacy concerns. Computers in Human Behavior, 25(1), 153–160. https://doi.org/10.1016/j.chb.2008.08.006
Fornell, C., & Larcker, D. F. (1981). Evaluating Structural Equation Models with Unobservable Variables and Measurement Error. Journal of Marketing Research, 18(1), 39–50. https://doi.org/10.1177/002224378101800104
Gerber, N., Gerber, P., & Volkamer, M. (2018). Explaining the privacy paradox: A systematic review of literature investigating privacy attitude and behavior. Computers & Security, 77, 226–261. https://doi.org/10.1016/j.cose.2018.04.002
Hair, J. F., Jr., Matthews, L. M., Matthews, R. L., & Sarstedt, M. (2017a). PLS-SEM or CB-SEM: Updated guidelines on which method to use. International Journal of Multivariate Data Analysis, 1(2), 107. https://doi.org/10.1504/ijmda.2017.10008574
Hair, J. F., Ringle, C. M., & Sarstedt, M. (2012). Editorial-Partial Least squares: The Better approach to structural equation modeling? Long Range Planning, 45(4–5), 312–319. https://doi.org/10.1016/j.lrp.2012.09.011
Hair, J. F., Risher, J. J., Sarstedt, M., & Ringle, C. M. (2019). When to use and how to report the results of PLS-SEM. European Business Review, 31(1), 2–24. https://doi.org/10.1108/EBR-11-2018-0203
Hair, J., Hollingsworth, C. L., Randolph, A. B., & Chong, A. Y. L. (2017b). An updated and expanded assessment of PLS-SEM in information systems research. Industrial management & data systems, 117(3), 442-458. https://doi.org/10.1108/IMDS-04-2016-0130
Hanif, Y., & Lallie, H. S. (2021). Security factors on the intention to use mobile banking applications in the UK older generation (55+). A mixed-method study using modified UTAUT and MTAM - with perceived cyber security, risk, and trust. Technology in Society, 67, 101693. https://doi.org/10.1016/j.techsoc.2021.101693
Hassaan, M., & Yaseen, A. (2024). Factors influencing customers’ adoption of mobile payment in Pakistan: Application of the extended meta-UTAUT model. Journal of Science and Technology Policy Management. https://doi.org/10.1108/JSTPM-01-2024-0029
Hayes, A. F., Montoya, A. K., & Rockwood, N. J. (2017). The analysis of mechanisms and their contingencies: PROCESS versus structural equation modeling. Australasian Marketing Journal, 25(1), 76–81. https://doi.org/10.1016/j.ausmj.2017.02.001
Henseler, J., Ringle, C. M., & Sinkovics, R. R. (2009). The use of partial lease squares path modeling in international marketing. Advances in International Marketing, 20 (2009), 277e319. https://doi.org/10.1108/S1474-7979(2009)0000020014
Introna, L. D. (2016). Algorithms, Governance, and Governmentality: On Governing Academic Writing. Science, Technology, & Human Values, 41(1), 17–49. https://doi.org/10.1177/0162243915587360
Jabbar, A., Geebren, A., Hussain, Z., Dani, S., & Ul-Durar, S. (2023). Investigating individual privacy within CBDC: A privacy calculus perspective. Research in International Business and Finance, 64, 101826. https://doi.org/10.1016/j.ribaf.2022.101826
Jain, S., Basu, S., Dwivedi, Y. K., & Kaur, S. (2022). Interactive voice assistants – Does brand credibility assuage privacy risks? Journal of Business Research, 139, 701–717. https://doi.org/10.1016/j.jbusres.2021.10.007
Jöreskog, K. G. (1971). Statistical analysis of sets of congeneric tests. Psychometrika, 36(2), 109-133. https://doi.org/10.1007/BF02291393
Jozani, M., Ayaburi, E., Ko, M., & Choo, K.-K. R. (2020). Privacy concerns and benefits of engagement with social media-enabled apps: A privacy calculus perspective. Computers in Human Behavior, 107, 106260. https://doi.org/10.1016/j.chb.2020.106260
Karizat, N., Delmonaco, D., Eslami, M., & Andalibi, N. (2021). Algorithmic Folk Theories and Identity: How TikTok Users Co-Produce Knowledge of Identity and Engage in Algorithmic Resistance. Proceedings of the ACM on Human-Computer Interaction, 5(CSCW2), 1–44. https://doi.org/10.1145/3476046
Kim & Kankanhalli. (2009). Investigating User Resistance to Information Systems Implementation: A Status Quo Bias Perspective. MIS Quarterly, 33(3), 567. https://doi.org/10.2307/20650309
Kim, Sodam, Park, Philip, & Yang, Sung-Byung. (2017). Influencing Factors on Users’ Resistance to the Mobile Easy Payment Services: Focusing on the Case of KakaoPay Users. Journal of Information Technology Services, 16(2), 139–156. https://doi.org/10.9716/KITS.2017.16.2.139
Kleinberg, J., Ludwig, J., Mullainathan, S., & Sunstein, C. R. (2018). Discrimination in the Age of Algorithms. Journal of Legal Analysis, 10, 113–174. https://doi.org/10.1093/jla/laz001
Knijnenburg, B. P., Kobsa, A., & Jin, H. (2013). Dimensionality of information disclosure behavior. International Journal of Human-Computer Studies, 71(12), 1144–1162. https://doi.org/10.1016/j.ijhcs.2013.06.003
Kock, N. (2015). Common Method Bias in PLS-SEM: A Full Collinearity Assessment Approach. International Journal of e-Collaboration, 11(4), 1–10. https://doi.org/10.4018/ijec.2015100101
Kokolakis, S. (2017). Privacy attitudes and privacy behaviour: A review of current research on the privacy paradox phenomenon. Computers & Security, 64, 122–134. https://doi.org/10.1016/j.cose.2015.07.002
Kucukusta, D., Law, R., Besbes, A., & Legohérel, P. (2015). Re-examining perceived usefulness and ease of use in online booking: The case of Hong Kong online users. International Journal of Contemporary Hospitality Management, 27(2), 185–198. https://doi.org/10.1108/IJCHM-09-2013-0413
Lau, J., Zimmerman, B., & Schaub, F. (2018). Alexa, Are You Listening?: Privacy Perceptions, Concerns and Privacy-seeking Behaviors with Smart Speakers. Proceedings of the ACM on Human-Computer Interaction, 2(CSCW), 1–31. https://doi.org/10.1145/3274371
Lee, H. (2020). Home IoT resistance: Extended privacy and vulnerability perspective. Telematics and Informatics, 49, 101377. https://doi.org/10.1016/j.tele.2020.101377
Lee, N., & Kwon, O. (2015). A privacy-aware feature selection method for solving the personalization–privacy paradox in mobile wellness healthcare services. Expert Systems with Applications, 42(5), 2764–2771. https://doi.org/10.1016/j.eswa.2014.11.031
Li, H., Sarathy, R. & Xu, H. (2010). Understanding Situational Online Information Disclosure as a Privacy Calculus. Journal of Computer Information Systems, 51(1), 62–71. https://doi.org/10.1080/08874417.2010.11645450
Li, L., Li, T., Cai, H., Zhang, J., & Wang, J. (2023). I will only know after using it: The repeat purchasers of smart home appliances and the privacy paradox problem. Computers & Security, 128, 103156. https://doi.org/10.1016/j.cose.2023.103156
Li, S., Mou, Y., & Xu, J. (2024). Disclosing Personal Health Information to Emotional Human Doctors or Unemotional AI Doctors? Experimental Evidence Based on Privacy Calculus Theory. International Journal of Human–Computer Interaction, 1–13. https://doi.org/10.1080/10447318.2024.2411619
Liu, Z., & Wang, X. (2018). How to regulate individuals’ privacy boundaries on social network sites: A cross-cultural comparison. Information & Management, 55(8), 1005–1023. https://doi.org/10.1016/j.im.2018.05.006
Lv, X., Chen, Y., & Guo, W. (2022). Adolescents’ Algorithmic Resistance to Short Video APP’s Recommendation: The Dual Mediating Role of Resistance Willingness and Resistance Intention. Frontiers in Psychology, 13, 859597. https://doi.org/10.3389/fpsyg.2022.859597
Mahmud, H., Islam, A. K. M. N., & Mitra, R. K. (2023). What drives managers towards algorithm aversion and how to overcome it? Mitigating the impact of innovation resistance through technology readiness. Technological Forecasting and Social Change, 193, 122641. https://doi.org/10.1016/j.techfore.2023.122641
Menon, D., & Shilpa, K. (2023). “Chatting with ChatGPT”: Analyzing the factors influencing users’ intention to Use the Open AI’s ChatGPT using the UTAUT model. Heliyon, 9(11), e20962. https://doi.org/10.1016/j.heliyon.2023.e20962
Merhi, M., Hone, K., & Tarhini, A. (2019). A cross-cultural study of the intention to use mobile banking between Lebanese and British consumers: Extending UTAUT2 with security, privacy and trust. Technology in Society, 59, 101151. https://doi.org/10.1016/j.techsoc.2019.101151
Migliore, G., Wagner, R., Cechella, F. S., & Liébana-Cabanillas, F. (2022). Antecedents to the Adoption of Mobile Payment in China and Italy: An Integration of UTAUT2 and Innovation Resistance Theory. Information Systems Frontiers, 24(6), 2099–2122. https://doi.org/10.1007/s10796-021-10237-2
Milberg, S. J., Smith, H. J., & Burke, S. J. (2000). Information Privacy: Corporate Management and National Regulation. Organization Science, 11(1), 35–57. https://doi.org/10.1287/orsc.11.1.35.12567
Möller, J., Trilling, D., Helberger, N., & Van Es, B. (2018). Do not blame it on the algorithm: An empirical assessment of multiple recommender systems and their impact on content diversity. Information, Communication & Society, 21(7), 959–977. https://doi.org/10.1080/1369118X.2018.1444076
Moore, G. C., & Benbasat, I. (1991). Development of an Instrument to Measure the Perceptions of Adopting an Information Technology Innovation. Information Systems Research, 2(3), 192–222. https://doi.org/10.1287/isre.2.3.192
Ooi, K.-B., Hew, J.-J., & Lin, B. (2018). Unfolding the privacy paradox among mobile social commerce users: A multi-mediation approach. Behaviour & Information Technology, 37(6), 575–595. https://doi.org/10.1080/0144929X.2018.1465997
Patil, P., Tamilmani, K., Rana, N. P., & Raghavan, V. (2020). Understanding consumer adoption of mobile payment in India: Extending Meta-UTAUT model with personal innovativeness, anxiety, trust, and grievance redressal. International Journal of Information Management, 54, 102144. https://doi.org/10.1016/j.ijinfomgt.2020.102144
Podsakoff, P. M., MacKenzie, S. B., Lee, J.-Y., & Podsakoff, N. P. (2003). Common method biases in behavioral research: A critical review of the literature and recommended remedies. Journal of Applied Psychology, 88(5), 879–903. https://doi.org/10.1037/0021-9010.88.5.879
Preacher, K. J., & Hayes, A. F. (2008). Asymptotic and resampling strategies for assessing and comparing indirect effects in multiple mediator models. Behavior research methods, 40(3), 879-891. https://doi.org/10.3758/BRM.40.3.879
Querci, I., Barbarossa, C., Romani, S., & Ricotta, F. (2022). Explaining how algorithms work reduces consumers’ concerns regarding the collection of personal data and promotes AI technology adoption. Psychology & Marketing, 39(10), 1888–1901. https://doi.org/10.1002/mar.21705
Rivis, A., Sheeran, P., & Armitage, C. J. (2006). Augmenting the theory of planned behaviour with the prototype/willingness model: Predictive validity of actor versus abstainer prototypes for adolescents’ health‐protective and health‐risk intentions. British Journal of Health Psychology, 11(3), 483–500. https://doi.org/10.1348/135910705X70327
Rogers, E. M. (1983). Diffusion of Innovations, The Free Press, New York, NY. https://ssrn.com/abstract=1496176
Schellewald, A. (2022). Theorizing “Stories About Algorithms” as a Mechanism in the Formation and Maintenance of Algorithmic Imaginaries. Social Media + Society, 8(1), 20563051221077025. https://doi.org/10.1177/20563051221077025
Schlosser, A. E. (2020). Self-disclosure versus self-presentation on social media. Current Opinion in Psychology, 31, 1–6. https://doi.org/10.1016/j.copsyc.2019.06.025
Shin, D. (2021). The effects of explainability and causability on perception, trust, and acceptance: Implications for explainable AI. International Journal of Human-Computer Studies, 146, 102551. https://doi.org/10.1016/j.ijhcs.2020.102551
Shin, D., & Park, Y. J. (2019). Role of fairness, accountability, and transparency in algorithmic affordance. Computers in Human Behavior, 98, 277–284. https://doi.org/10.1016/j.chb.2019.04.019
Shin, D., Kee, K. F., & Shin, E. Y. (2022a). Algorithm awareness: Why user awareness is critical for personal privacy in the adoption of algorithmic platforms? International Journal of Information Management, 65, 102494. https://doi.org/10.1016/j.ijinfomgt.2022.102494
Shin, D., Rasul, A., & Fotiadis, A. (2022b). Why am I seeing this? Deconstructing algorithm literacy through the lens of users. Internet Research, 32(4), 1214–1234. https://doi.org/10.1108/INTR-02-2021-0087
Shin, D., Zhong, B., & Biocca, F. A. (2020). Beyond user experience: What constitutes algorithmic experiences? International Journal of Information Management, 52, 102061. https://doi.org/10.1016/j.ijinfomgt.2019.102061
Siles, I., Segura-Castillo, A., Solís, R., & Sancho, M. (2020). Folk theories of algorithmic recommendations on Spotify: Enacting data assemblages in the global South. Big Data & Society, 7(1), 205395172092337. https://doi.org/10.1177/2053951720923377
Sommestad, T., Karlzén, H., & Hallberg, J. (2019). The Theory of Planned Behavior and Information Security Policy Compliance. Journal of Computer Information Systems, 59(4), 344–353. https://doi.org/10.1080/08874417.2017.1368421
Song, B., Xu, H., Hu, W., Li, Y., & Guo, Y. (2024). How to calculate privacy: Privacy concerns and service robots’ use intention in hospitality. Current Issues in Tourism, 27(21), 3401–3417. https://doi.org/10.1080/13683500.2023.2265029
Taddicken, M. (2014). The ‘Privacy Paradox’ in the Social Web: The Impact of Privacy Concerns, Individual Characteristics, and the Perceived Social Relevance on Different Forms of Self-Disclosure. Journal of Computer-Mediated Communication, 19(2), 248–273. https://doi.org/10.1111/jcc4.12052
Tamilmani, K., Rana, N. P., & Dwivedi, Y. K. (2021). Consumer Acceptance and Use of Information Technology: A Meta-Analytic Evaluation of UTAUT2. Information Systems Frontiers, 23(4), 987–1005. https://doi.org/10.1007/s10796-020-10007-6
Taylor, S., & Todd, P. A. (1995). Understanding Information Technology Usage: A Test of Competing Models. Information Systems Research, 6(2), 144–176. https://doi.org/10.1287/isre.6.2.144
Thompson, R. L., Higgins, C. A., & Howell, J. M. (1991). Personal Computing: Toward a Conceptual Model of Utilization. MIS Quarterly, 15(1), 125. https://doi.org/10.2307/249443
Trepte, S., Reinecke, L., Ellison, N. B., Quiring, O., Yao, M. Z., & Ziegele, M. (2017). A Cross-Cultural Perspective on the Privacy Calculus. Social Media + Society, 3(1), 2056305116688035. https://doi.org/10.1177/2056305116688035
Tufekci, Z. (2008). Can You See Me Now? Audience and Disclosure Regulation in Online Social Network Sites. Bulletin of Science, Technology & Society, 28(1), 20–36. https://doi.org/10.1177/0270467607311484
Velkova, J., & Kaun, A. (2021). Algorithmic resistance: Media practices and the politics of repair. Information, Communication & Society, 24(4), 523–540. https://doi.org/10.1080/1369118X.2019.1657162
Venkatesh, Morris, Davis, & Davis. (2003). User Acceptance of Information Technology: Toward a Unified View. MIS Quarterly, 27(3), 425. https://doi.org/10.2307/30036540
Venkatesh, Thong, & Xu. (2012). Consumer Acceptance and Use of Information Technology: Extending the Unified Theory of Acceptance and Use of Technology. MIS Quarterly, 36(1), 157. https://doi.org/10.2307/41410412
Vimalkumar, M., Sharma, S. K., Singh, J. B., & Dwivedi, Y. K. (2021). ‘Okay google, what about my privacy?’: User’s privacy perceptions and acceptance of voice based digital assistants. Computers in Human Behavior, 120, 106763. https://doi.org/10.1016/j.chb.2021.106763
Wang, X., Wuji, S., Liu, Y., Luo, R., & Qiu, C. (2024). Study on the impact of recommendation algorithms on user perceived stress and health management behaviour in short video platforms. Information Processing & Management, 61(3), 103674. https://doi.org/10.1016/j.ipm.2024.103674
Widyanto, H. A., Kusumawardani, K. A., & Yohanes, H. (2022). Safety first: Extending UTAUT to better predict mobile payment adoption by incorporating perceived security, perceived risk and trust. Journal of Science and Technology Policy Management, 13(4), 952–973. https://doi.org/10.1108/JSTPM-03-2020-0058
Wu, W., Huang, X., & Li, X. (2023). Technology moral sense: Development, reliability, and validity of the TMS scale in Chinese version. Frontiers in Psychology, 14, 1056569. https://doi.org/10.3389/fpsyg.2023.1056569
Yang, M., Al Mamun, A., Gao, J., Rahman, M. K., Salameh, A. A., & Alam, S. S. (2024). Predicting m-health acceptance from the perspective of unified theory of acceptance and use of technology. Scientific Reports, 14(1), 339. https://doi.org/10.1038/s41598-023-50436-2
Ying, S., Huang, Y., Qian, L., & Song, J. (2023). Privacy paradox for location tracking in mobile social networking apps: The perspectives of behavioral reasoning and regulatory focus. Technological Forecasting and Social Change, 190, 122412. https://doi.org/10.1016/j.techfore.2023.122412
Ytre-Arne, B., & Moe, H. (2021). Folk theories of algorithms: Understanding digital irritation. Media, Culture & Society, 43(5), 807–824. https://doi.org/10.1177/0163443720972314
Zhang, D., Boerman, S. C., Hendriks, H., van der Goot, M. J., Araujo, T. & Voorveld, H. (2024a). “They Know Everything”: Folk Theories, Thoughts, and Feelings About Dataveillance in Media Technologies. International Journal of Communication, 18, 21. Rereieved from https://ijoc.org/index.php/ijoc/article/ view/21495
Zhang, J., & Han, T. (2023). Individualism and collectivism orientation and the correlates among Chinese college students. Current Psychology, 42(5), 3811–3821. https://doi.org/10.1007/s12144-021-01735-2
Zhang, L., Bi, W., Zhang, N., & He, L. (2024b). Coping with Homogeneous Information Flow in Recommender Systems: Algorithmic Resistance and Avoidance. International Journal of Human–Computer Interaction, 40(22), 6899–6912. https://doi.org/10.1080/10447318.2023.2267931
Zhang, X., & Zhang, Z. (2024). Leaking my face via payment: Unveiling the influence of technology anxiety, vulnerabilities, and privacy concerns on user resistance to facial recognition payment. Telecommunications Policy, 48(3), 102703. https://doi.org/10.1016/j.telpol.2023.102703
Zhou, S., & Liu, Y. (2023). Effects of Perceived Privacy Risk and Disclosure Benefits on the Online Privacy Protection Behaviors among Chinese Teens. Sustainability, 15(2), 1657. https://doi.org/10.3390/su15021657
Zhu, H., Ou, C. X. J., Van Den Heuvel, W. J. A. M., & Liu, H. (2017). Privacy calculus and its utility for personalization services in e-commerce: An analysis of consumer decision-making. Information & Management, 54(4), 427–437. https://doi.org/10.1016/j.im.2016.10.001
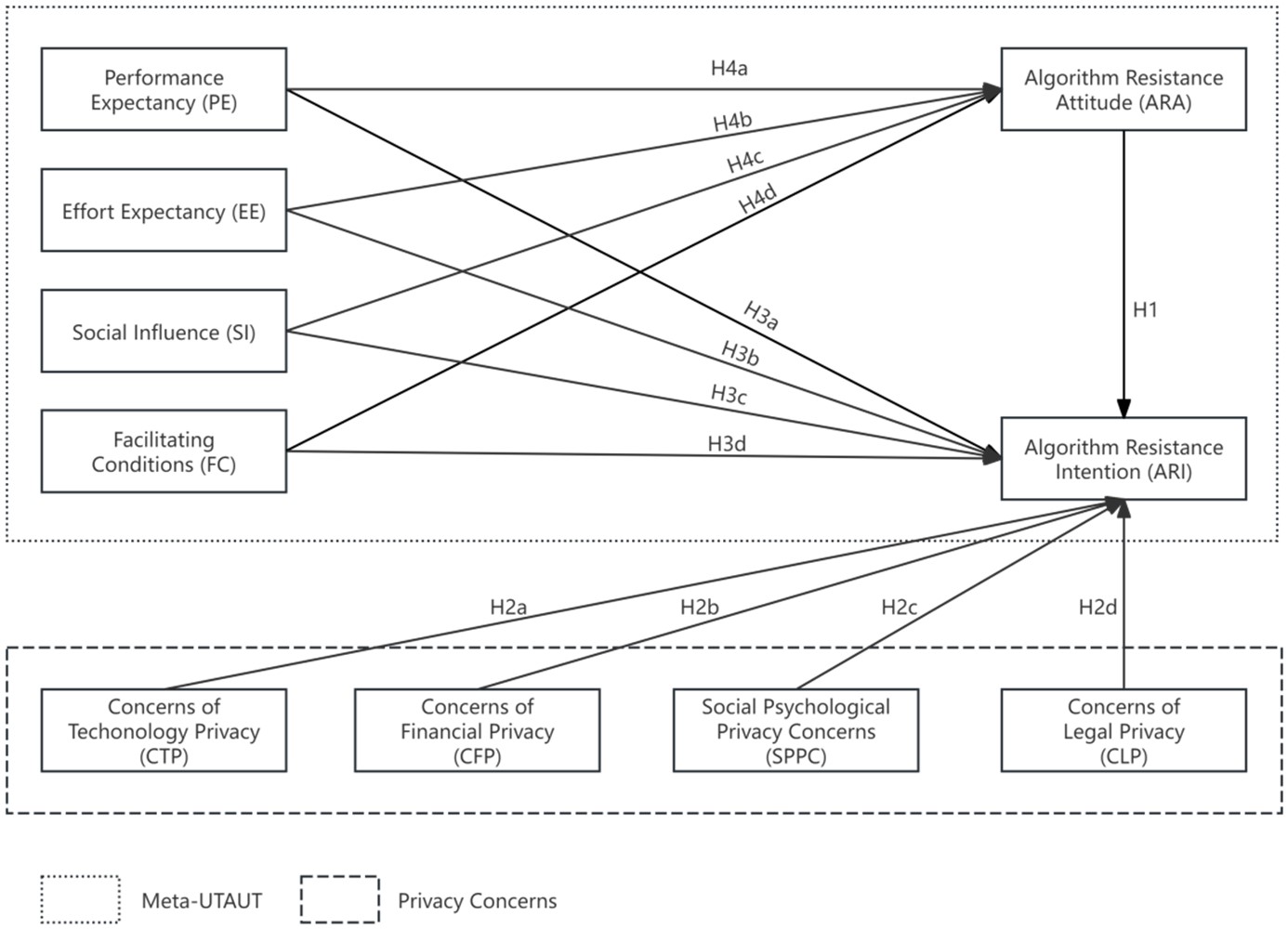

This work is licensed under a Creative Commons Attribution 4.0 International License.
Copyright for this article is retained by the author(s), with first publication rights granted to the journal.
This is an open-access article distributed under the terms and conditions of the Creative Commons Attribution license (http://creativecommons.org/licenses/by/4.0/).