Application of Machine Vision in Structural Deformation and Health Monitoring
Abstract
There are two main methods of structural health monitoring. Among them, the traditional structure health monitoring technology is mainly artificial, which has some problems such as inflexibility, large error, high cost and difficult to adapt to the change of natural environment. The structural health monitoring method based on machine vision has the characteristics of flexible measuring point, high accuracy, fast speed and no contact. A variety of machine vision technologies, such as image acquisition, image processing, three-dimensional vision and deep learning technologies, have made great progress, and their application scenarios are constantly expanding. This paper is based on infrared image acquisition technology, 3D vision acquisition technology, image stitching technology, stereo vision technology four perspectives. The improvement of machine vision technology in the field of structural health monitoring is described in detail. The development trend of machine vision acquisition technology and image processing technology and the huge growth space of machine vision technology are analyzed. The application range of these key technologies is also introduced. The application results in building crack detection, seepage detection and fire detection are summarized, and the future development of this technology is prospected from the aspects of algorithm robustness.
References
[2] Abedi, M., & Naser, M. Z. (2021). Rapid, autonomous and intelligent machine learning approach to identify fire-vulnerable bridges. Applied Soft Computing, 113, 1514–1516. https://doi.org/10.1016/j.asoc.2021.107964
[3] Bai, Y., Zhang, B., Xu, N., Zhou, J., Shi, J., & Diao, Z. (2023). Vision-based navigation and guidance for agricultural autonomous vehicles and robots: A review. Computers and Electronics in Agriculture, 61(5), 673–681. https://doi.org/10.1016/j.compag.2023.107048
[4] Brown, M., & Lowe, D. G. (2007). Automatic panoramic image stitching using invariant features. International Journal of Computer Vision, 74(1), 59–73. https://doi.org/10.1007/s11263-006-0002-3
[5] Cardellicchio, A., Ruggieri, S., Nettis, A., Reno, V., & Uva, G. (2023). Physical interpretation of machine learning-based recognition of defects for the risk management of existing bridge heritage. Engineering Failure Analysis, 9(2–3), 81–92. https://doi.org/10.1016/j.engfailanal.2023.106183
[6] Fossum, E. R., & Hondongwa, D. B. (2014). A review of the pinned photodiode for CCD and CMOS image sensors. IEEE Journal of the Electron Devices Society, 2(3), 33–43. https://doi.org/10.1109/JEDS.2014.2306412
[7] Gade, R., & Moeslund, T. B. (2014). Thermal cameras and applications: A survey. Machine Vision and Applications, 25(1), 245–262. https://doi.org/10.1007/s00138-013-0570-5
[8] Hosseinzadeh, A. Z., Tehrani, M. H., & Harvey, P. S., Jr. (2020). Modal identification of building structures using vision-based measurements from multiple interior surveillance cameras. Engineering Structures, 99(5), 752–757. https://doi.org/10.1016/j.engstruct.2020.109058
[9] Ker, J., Wang, L., Rao, J., & Lim, T. (2018). Deep learning applications in medical image analysis. IEEE Access, 6, 9375–9389. https://doi.org/10.1109/ACCESS.2017.2788044
[10] Liu, Y. (2022). Improved feature point pair purification algorithm based on SIFT during endoscope image stitching. Frontiers in Neurorobotics, 16, 81–92. https://doi.org/10.3389/fnbot.2022.888627
[11] Laga, H., Jospin, L. V., Boussaid, F., & Bennamoun, M. (2022). A survey on deep learning techniques for stereo-based depth estimation. IEEE Transactions on Pattern Analysis and Machine Intelligence, 44(4), 1738–1764. https://doi.org/10.1109/TPAMI.2021.3079303
[12] Spencer, B. F., Jr., Hoskere, V., & Narazaki, Y. (2019). Advances in computer vision-based civil infrastructure inspection and monitoring. Engineering, 5(2), 199–222. https://doi.org/10.1016/j.eng.2018.11.030
[13] Sirmacek, B., Hoegner, L., & Stilla, U. (2011). Detection of windows and doors from thermal images by grouping geometrical features. 2011 Proceedings of Joint Urban Remote Sensing Event (JURSE 2011), 133–136. https://doi.org/10.1109/JURSE.2011.5764752
[14] Si, Y., Rouse, J. P., & Hyde, C. J. (2020). Potential difference methods for measuring crack growth: A review. International Journal of Fatigue, 28, 59–63. https://doi.org/10.1016/j.ijfatigue.2019.105305
[15] Sidahmed, M. A., & Boraie, M. T. (1990). Dual camera calibration for 3-D machine vision metrology. IEEE Transactions on Instrumentation and Measurement, 39(3), 512–516. https://doi.org/10.1109/19.54860
[16] Tsai, R. Y. (1987). A versatile camera calibration technique for high-accuracy 3D machine vision metrology using off-the-shelf TV cameras and lenses. IEEE Journal of Robotics and Automation, 3(4), 323–344. https://doi.org/10.1109/JRA.1987.1087109
[17] Tan, Y., Li, X., Lai, J., & Ai, J. (2024). Real-time tunnel lining leakage image semantic segmentation via multiple attention mechanisms. Measurement Science and Technology, 35(7). https://doi.org/10.1088/1361-6501/ad174e
[18] Wulff, A. M., Hashida, T., Watanabe, K., & Takahashi, H. (1999). Attenuation behaviour of tuffaceous sandstone and granite during microfracturing. Geophysical Journal International, 139(2), 395–409. https://doi.org/10.1046/j.1365-246X.1999.00939.x
[19] Wang, Z. F., et al. (2022). Convolutional neural-network-based automatic dam-surface seepage defect identification from thermograms collected from UAV-mounted thermal imaging camera. Construction and Building Materials, 323(14), 59–63. https://doi.org/10.1016/j.conbuildmat.2022.126548
[20] Yu, Z., Li, L., Xie, J., Wang, C., Li, W., & Ning, X. (2024). Pedestrian 3D shape understanding for person re-identification via multi-view learning. IEEE Transactions on Circuits and Systems for Video Technology, 34(7), 5589–5602. https://doi.org/10.1109/TCSVT.2023.3327152
[21] Zhang, Z. Y. (2000). A flexible new technique for camera calibration. IEEE Transactions on Pattern Analysis and Machine Intelligence, 22(11), 1330–1334. https://doi.org/10.1109/34.888718
[22] Zhao, J. X., Xu, H., Liu, H. C., Wu, J. Q., Zheng, Y. C., & Wu, D. Y. (2019). Detection and tracking of pedestrians and vehicles using roadside LiDAR sensors. Transportation Research Part C: Emerging Technologies, 100, 68–87. https://doi.org/10.1016/j.trc.2019.01.018
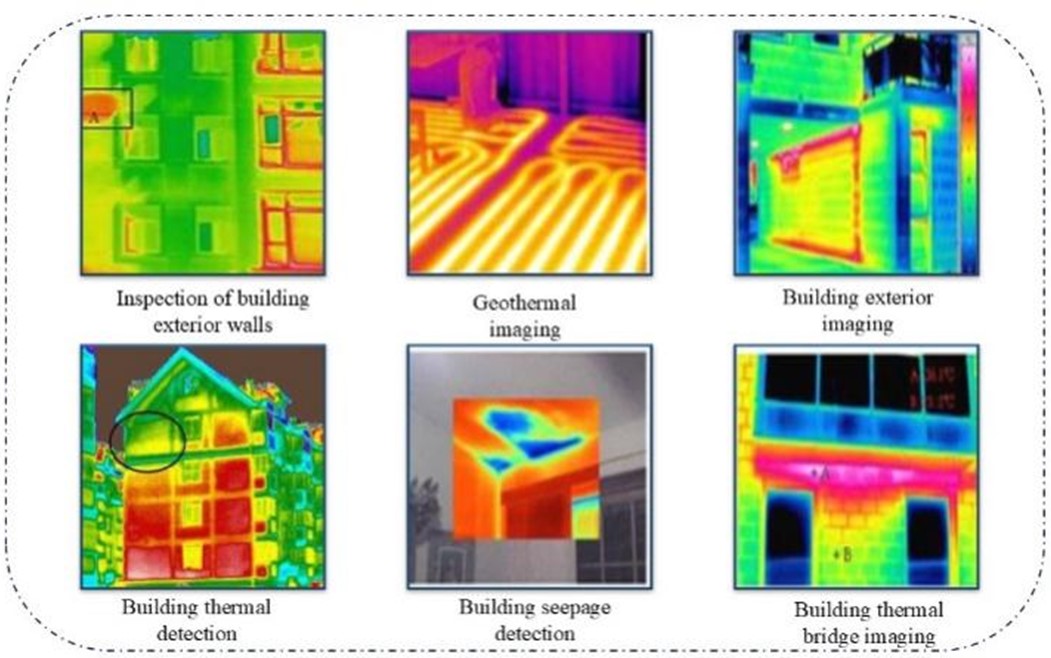

This work is licensed under a Creative Commons Attribution 4.0 International License.
Copyright for this article is retained by the author(s), with first publication rights granted to the journal.
This is an open-access article distributed under the terms and conditions of the Creative Commons Attribution license (http://creativecommons.org/licenses/by/4.0/).