Adaptive Containment Control for Nonlinear Multi-Agent Systems with Input Quantization and Sensor Faults
Abstract
This study investigates the adaptive containment control problem for a class of nonlinear multi-agent systems with input quantization and sensor faults. A state observer and a radial basis function neural network are respectively employed to estimate unmeasurable states and approximate unknown nonlinear functions. An absolute cubic Lyapunov function is designed to compensate for the influence of sensor faults on the systems. A filter is introduced to reduce computational complexity. Adaptive laws are developed to update the estimates of uncertain dynamic parameters, fault coefficients, and the filter-error compensation term. A distributed adaptive control scheme is proposed to ensure that all followers converge to the convex hull formed by the leaders. The stability of the closed-loop system is strictly proved based on stability theory, and the effectiveness of the proposed control method is verified by numerical simulation.
References
[2] Yang, J., Zhang, J., & Wang, H. (2021). Urban traffic control in software defined internet of things via a multi-agent deep reinforcement learning approach. IEEE Transactions on Intelligent Transportation Systems, 22(6), 3742–3754. https://doi.org/10.1109/TITS.2020.3023788
[3] Parrany, A. M., & Alasty, A. (2025). Decentralized leader-following control of a heterogeneous swarm robotic system subject to network-induced imperfections. Unmanned Systems, 13(01), 69–90. https://doi.org/10.1142/S2301385025500050
[4] Cai, J., Wu, W., Yi, C., et al. (2025). Neural adaptive dynamic event-triggered containment control for uncertain multi-agent systems under Markovian switching dynamics. Cognitive Computation, 17(1), 1–14. https://doi.org/10.1007/s12559-024-10388-9
[5] Habibi, H., Iftekhar, L., Rahman, M. H., et al. (2025). Cooperative learning‐based practical formation‐containment control with prescribed performance for heterogeneous clusters of UAV/USV. Asian Journal of Control, 27(2), 921–947. https://doi.org/10.1002/asjc.3480
[6] Wang, H., & Li, M. (2025). Adaptive fuzzy nonsingular fixed-time safety flight containment control for multi-UAVs. IEEE Transactions on Aerospace and Electronic Systems, 61(1), 802–812. https://doi.org/10.1109/TAES.2024.3448398
[7] Zhang, T., Zhang, S., Li, H., et al. (2025). Velocity-free prescribed-time orbit containment control for satellite clusters under actuator saturation. Advances in Space Research, 75(6), 5110–5123. https://doi.org/10.1016/j.asr.2024.12.039
[8] Zhang, Z., Huang, B., Zhou, X., et al. (2025). Fully distributed dynamic event-triggered formation-containment control for networked unmanned surface vehicles with intermittent wireless network communications. ISA Transactions, 156, 202–216. https://doi.org/10.1016/j.isatra.2024.10.033
[9] Wang, L., & Dong, J. (2022). Adaptive fuzzy consensus tracking control for uncertain fractional-order multiagent systems with event-triggered input. IEEE Transactions on Fuzzy Systems, 30(2), 310–320. https://doi.org/10.1109/TFUZZ.2020.3037957
[10] Yao, D., Wu, Y., Ren, H., et al. (2025). Event-based adaptive sliding-mode containment control for multiple networked mechanical systems with parameter uncertainties. IEEE Transactions on Automation Science and Engineering, 22, 264–275. https://doi.org/10.1109/TASE.2024.3349634
[11] Wang, L., Yan, H., Hu, X., et al. (2025). Fixed-time bipartite containment control for heterogeneous multiagent systems under DoS attacks: An event-triggered mechanism. IEEE Transactions on Systems, Man, and Cybernetics: Systems, 55(4), 2782–2794. https://doi.org/10.1109/TSMC.2024.3523705
[12] Ba, D., Li, Y., & Tong, S. (2019). Fixed-time adaptive neural tracking control for a class of uncertain nonstrict nonlinear systems. Neurocomputing, 363, 273–280. https://doi.org/10.1016/j.neucom.2019.06.063
[13] Ning, J., Wang, Y., Wang, E., et al. (2025). Fuzzy trajectory tracking control of under-actuated unmanned surface vehicles with ocean current and input quantization. IEEE Transactions on Systems, Man, and Cybernetics: Systems, 55(1), 63–72. https://doi.org/10.1109/TSMC.2024.3460370
[14] Li, H., & Li, M. (2025). A dynamic gain method to event-triggered tracking control for uncertain nonlinear systems with input quantization. IEEE Transactions on Automatic Control, 1–8. https://doi.org/10.1109/TAC.2025.3545551
[15] Yang, Y., Sui, S., Liu, T., et al. (2025). Adaptive predefined time control for stochastic switched nonlinear systems with full-state error constraints and input quantization. IEEE Transactions on Cybernetics, 1–12. https://doi.org/10.1109/TCYB.2025.3555205
[16] Cheng, W., Cheng, H., Wang, F., et al. (2025). Fixed-time neural consensus control for nonlinear multiagent systems with state and input quantization. Chaos, Solitons & Fractals, 194, 116145. https://doi.org/10.1016/j.chaos.2025.116145
[17] Sui, S., Zhao, L., & Chen, C. P. (2024). Adaptive fuzzy predefined-time tracking control design for nonstrict-feedback high-order nonlinear systems with input quantization. IEEE Transactions on Fuzzy Systems, 32(10), 5978–5990. https://doi.org/10.1109/TFUZZ.2024.3431047
[18] Wu, J., Yang, Y., Chen, W., et al. (2025). Adaptive neural fault tolerant control for input-delayed stochastic systems subject to states and input quantization. IEEE Transactions on Systems, Man, and Cybernetics: Systems, 55(4), 2451–2462. https://doi.org/10.1109/TSMC.2025.3525508
[19] Xia, X., Li, C., Zhang, T., et al. (2025). Adaptive prescribed performance optimal control for strict-feedback nonlinear systems with input delay and input quantization. Journal of the Franklin Institute, 362(4), 107568. https://doi.org/10.1016/j.jfranklin.2025.107568
[20] Qin, L., He, X., Yan, R., et al. (2017). Active fault-tolerant control for a quadrotor with sensor faults. Journal of Intelligent & Robotic Systems, 88, 449–467. https://doi.org/10.1007/s10846-017-0474-0
[21] Nadi, A., Mahzoon, M., & Azadi Yazdi, E. (2025). Adaptive fault tolerant control for cantilever thick plates with piezoelectric patches. Scientific Reports, 15(1), 388. https://doi.org/10.1038/s41598-024-84420-1
[22] Ri, K. C., Kim, Y. I., & Ri, S. J. (2025). Robust fault-tolerant control and application in DCS with uncertainty and time delay. International Journal of Dynamics and Control, 13(2), 44. https://doi.org/10.1007/s40435-024-01535-z
[23] Wu, T., Yu, Z., & Li, S. (2022). Observer-based adaptive fuzzy quantized fault-tolerant control of nonstrict-feedback nonlinear systems with sensor fault. IEEE Transactions on Fuzzy Systems, 31(6), 1900–1911. https://doi.org/10.1109/TFUZZ.2022.3216113
[24] Ghoreishee, A., & Soroush, M. (2025). Adaptive fault-tolerant observer-based control for multi-input multi-output interconnected systems with bandwidth-limited communication. Control Engineering Practice, 156, 106217. https://doi.org/10.1016/j.conengprac.2024.106217
[25] Liu, X., Yan, M., Yang, P., et al. (2024). Unknown input observer based neuro-adaptive fault-tolerant control for vehicle platoons with sensor fault and output quantization. Control Engineering Practice, 150, 106007. https://doi.org/10.1016/j.conengprac.2024.106007
[26] Fang, X., Fan, H., Liu, L., et al. (2023). Decentralized adaptive fault-tolerant control of interconnected systems with sensor faults. Journal of Control and Decision, 12(2), 289–305. https://doi.org/10.1080/23307706.2023.2227184
[27] Ye, Z., Yu, Z., Jiang, B., et al. (2025). Event-triggered fault-tolerant tracking control for multiagent systems under actuator/sensor faults. ISA Transactions, 156, 30–38. https://doi.org/10.1016/j.isatra.2024.11.012
[28] Lv, W., Lu, J., Li, Y., et al. (2022). Adaptive neural finite-time control of nonlinear systems subject to sensor hysteresis. Journal of the Franklin Institute, 359(7), 2932–2948. https://doi.org/10.1016/j.jfranklin.2022.02.032
[29] Polycarpou, M. M., & Ioannou, P. A. (1996). A robust adaptive nonlinear control design. Automatica, 32(3), 423–427. https://doi.org/10.1016/0005-1098(95)00147-6
[30] Deng, H., & Krstić, M. (1997). Stochastic nonlinear stabilization—I: A backstepping design. Systems & Control Letters, 32(3), 143–150. https://doi.org/10.1016/S0167-6911(97)00068-6
[31] Hou, Z., Cheng, L., & Tan, M. (2009). Decentralized robust adaptive control for the multiagent system consensus problem using neural networks. IEEE Transactions on Systems, Man, and Cybernetics, Part B (Cybernetics), 39(3), 636–647. https://doi.org/10.1109/TSMCB.2008.2007810
[32] Wang, X., Chen, Z., & Yang, G. (2007). Finite-time-convergent differentiator based on singular perturbation technique. IEEE Transactions on Automatic Control, 52(9), 1731–1737. https://doi.org/10.1109/TAC.2007.904290
[33] Xing, L., Wen, C., Zhu, Y., et al. (2016). Output feedback control for uncertain nonlinear systems with input quantization. Automatica, 65, 191–202. https://doi.org/10.1016/j.automatica.2015.11.028
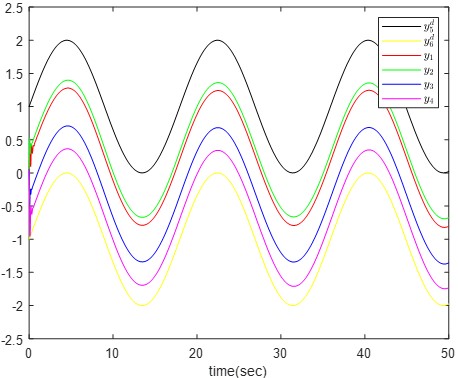

This work is licensed under a Creative Commons Attribution 4.0 International License.
Copyright for this article is retained by the author(s), with first publication rights granted to the journal.
This is an open-access article distributed under the terms and conditions of the Creative Commons Attribution license (http://creativecommons.org/licenses/by/4.0/).