Ergodic Foundations of Langevin-Based MCMC
Abstract
In this work, we provide a comprehensive theoretical analysis of Langevin diffusion and its applications to Markov Chain Monte Carlo (MCMC) methods. We establish the ergodicity of continuous-time Langevin diffusion processes, proving their convergence to target distributions under suitable regularity conditions. The analysis is then extended to discrete-time settings, examining the properties of the Unadjusted Langevin Algorithm (ULA) and the Metropolis-Adjusted Langevin Algorithm (MALA). Employing tools from stochastic processes, ergodic theory, and Markov chain theory, we establish strong convergence results using Foster-Lyapunov drift conditions, coupling arguments, and geometric ergodicity. The paper explores connections between Langevin diffusion and optimal transport theory, highlighting recent developments in adaptive methods, transport map accelerated MCMC, and applications to high-dimensional Bayesian inference. Our theoretical results provide insights into algorithm design, parameter tuning, and convergence diagnostics for Langevin-based MCMC methods, bridging the gap between theory and practice in the development of efficient sampling algorithms for complex probability distributions.
References
[2] Durmus, A., & Moulines, E. (2017). Nonasymptotic convergence analysis for the unadjusted langevin algorithm. The Annals of Applied Probability, 27, 1551-1587, 06/2017. https://doi.org/10.1214/16-aap1238.
[3] Roberts, G. O., & Rosenthal, J. S. (2009). Examples of adaptive mcmc. Journal of Computational and Graphical Statistics, 18, 349-367. 01/2009. https://doi.org/10.1198/jcgs.2009.06134.
[4] H. Kaspi, S. P. Meyn, and R. L. Tweedie, (1997). Markov chains and stochastic stability. Journal of the American Statistical Association, 92, p. 792, 06/1997. https://doi.org/10.2307/2965732
[5] Chen, Y., Georgiou, T. T., & Pavon, M. (2015). On the relation between optimal transport and schrodinger bridges: A stochastic control viewpoint. Journal of Optimization Theory and Applications, 169, 671-691, 09/2015. https://doi.org/10.1007/s10957-015-0803-z
[6] Parno, M. D., & Marzouk, Y. M. (2018). Transport map accelerated markov chain monte carlo. SIAM/ASA Journal on Uncertainty Quantification, 6, 645-682, 01/2018.https://doi.org/10.1137/17m1134640
[7] Roberts, G. O., & Tweedie, R. L. (1996). Exponential convergence of langevin distributions and their discrete approximations. Bernoulli, 2, p.341, 12/1996. https://doi.org/10.2307/3318418
[8] Dalalyan, A. S. (2014). Theoretical guarantees for approximate sampling from smooth and log-concave densities. arXiv (Cornell University), 01/2014. https://doi.org/10.48550/arxiv.1412.7392
[9] Cheng, X., and Bartlett, P. (2017). Convergence of langevin mcmc in kl-divergence. arXiv(Cornell University), 01/2017. https://doi.org/10.48550/arxiv.1705.09048
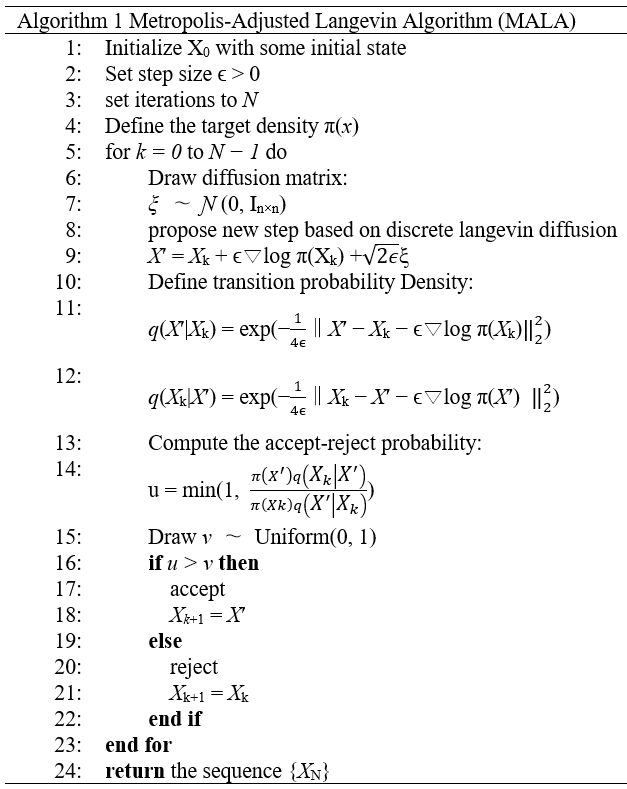

This work is licensed under a Creative Commons Attribution 4.0 International License.
Copyright for this article is retained by the author(s), with first publication rights granted to the journal.
This is an open-access article distributed under the terms and conditions of the Creative Commons Attribution license (http://creativecommons.org/licenses/by/4.0/).