Study On the Detection of Dry Matter in Silage Corn Feed Based on Near Infrared Spectroscopy
Abstract
This study explored the application of a portable near-infrared (NIR) spectrometer for analyzing silage corn feed quality, specifically focusing on developing a quantitative detection model for dry matter content. Spectral data were collected within the 855-1890 nm range using a portable NIR spectrometer, and the dataset was partitioned into calibration and prediction sets using the SPXY algorithm. An Extreme Learning Machine (ELM) model optimized by Particle Swarm Optimization (PSO) was employed for modeling. Five preprocessing methods were evaluated: Moving Average Filter (MAF), Savitzky-Golay Filter (SGF), Multiplicative Scatter Correction (MSC), Standard Normal Variate (SNV) transformation, and First Derivative (FD). To enhance model performance, feature wavelengths were selected using three methods: Bootstrap Soft Shrinkage (BOSS), Competitive Adaptive Reweighted Sampling (CARS), and Iterative Retained Information Variable (IRIV). The optimal model combining SNV preprocessing with BOSS feature selection achieved a prediction correlation coefficient () of 0.8708 and Root Mean Square Error of Prediction (RMSEP) of 0.6802. These results demonstrate the potential of portable NIR spectroscopy for rapid dry matter content determination in silage corn feed.
References
Büning-Pfaue, H. (2003). Analysis of water in food by near infrared spectroscopy. Food Chemistry, 82(1), 107–115. https://doi.org/10.1016/S0308-8146(02)00583-6
Deng, B. C., Yun, Y. H., Cao, D. S., Yin, Y. L., Wang, W. T., Lu, H. M., Luo, Q. Y., & Liang, Y. Z. (2016). A bootstrapping soft shrinkage approach for variable selection in chemical modeling. Analytica Chimica Acta, 908, 63–74. https://doi.org/10.1016/j.aca.2016.01.001
Fuglestad, E. M., Vik, J., Finstad, T., & Soraa, R. A. (2021). Compressed growth—The transforming power of the round bale technology. Journal of Rural Studies, 84, 174–179. https://doi.org/10.1016/j.jrurstud.2021.04.005
García-Chávez, I., Meraz-Romero, E., Castelán-Ortega, O., Zaragoza Esparza, J., Osorio Avalos, J., Robles Jimenez, L. E., & González-Ronquillo, M. (2020). Corn silage, meta-analysis of the quality and yield of different regions in the world. Preprints. https://doi.org/10.20944/preprints202010.0094.v1
Huang, W. C., Liu, H. Y., Zhang, Y., Mi, R. W., Tong, C. G., Xiao, W., & Shuai, B. (2021). Railway dangerous goods transportation system risk identification: Comparisons among SVM, PSO-SVM, GA-SVM and GS-SVM. Applied Soft Computing, 109, Article 107541. https://doi.org/10.1016/j.asoc.2021.107541
Li, H. D., Liang, Y. Z., Xu, Q. S., & Cao, D. S. (2009). Key wavelengths screening using competitive adaptive reweighted sampling method for multivariate calibration. Analytica Chimica Acta, 648(1), 77–84. https://doi.org/10.1016/j.aca.2009.06.046
Li, H. D., Xe, Q. S., & Liang, Y. Z. (2018). libPLS: An integrated library for partial least squares regression and linear discriminant analysis. Chemometrics and Intelligent Laboratory Systems, 176, 34–43. https://doi.org/10.1016/j.chemolab.2018.03.003
Liu, L. H., Zareef, M., Wang, Z., Li, H. Q., Chen, Q. S., & Ouyang, Q. (2023). Monitoring chlorophyll changes during Tencha processing using portable near-infrared spectroscopy. Food Chemistry, 412, Article 135505. https://doi.org/10.1016/j.foodchem.2023.135505
Ma, Y., Zhang, G. Z., & Rita-Cindy, S. A. A. (2019). Quantification of water, protein and soluble sugar in mulberry leaves using a handheld near-infrared spectrometer and multivariate analysis. Molecules, 24(24), Article 4439. https://doi.org/10.3390/molecules24244439
Ribas, T. M. B., Neumann, M., Horst, E. H., Cristo, F. B., Moresco, E. M., & de Almeida, E. R. (2021). Effect of inoculants in corn silage on dry matter digestibility, ingestive behavior, performance and carcass of heifers. Semina: Ciências Agrárias, 42(3), 1271–1285. https://doi.org/10.5433/1679-0359.2021v42n3p1271
Wang, M. C., Zhang, X. Y., Niu, X. F., Wang, F. Y., Xu, Z. S., Zhang, X. Q., Chen, S. B., Wang, M. C., & IEEE. (2018, August 19–20). Inversion of heavy metal content in a copper mining area based on extreme learning machine optimized by particle swarm algorithm. In 10th IAPR Workshop on Pattern Recognition in Remote Sensing (PRRS), Beijing, China.
Wu, J., Bai, Q. L., Su, S. B., Chen, S. J., Meng, Q. X., & Yan, Y. L. (2005). Near-infrared reflectance spectroscopy analysis of cellulose content in corn stalk. Chinese Journal of Analytical Chemistry, 33(10), 1421–1423.
Yin, X. T., Wang, P. F., Yan, Z. Q., Yang, Q. L., Huang, X. Y., & Gun, S. (2024). Effects of whole-plant corn silage on growth performance, serum biochemical indices, and fecal microorganisms in Hezuo pigs. Animals, 14(5), Article 662. https://doi.org/10.3390/ani14050662
Yun, Y. H., Wang, W. T., Tan, M. L., Liang, Y. Z., Li, H. D., Cao, D. S., Lu, H. M., & Xu, Q. S. (2014). A strategy that iteratively retains informative variables for selecting optimal variable subset in multivariate calibration. Analytica Chimica Acta, 807, 36–43. https://doi.org/10.1016/j.aca.2013.11.032
Zhang, M. Q., Zhao, C., Shao, Q. J., Yang, Z. D., Zhang, X. F., Xu, X. F., & Hassan, M. (2019). Determination of water content in corn stover silage using near-infrared spectroscopy. International Journal of Agricultural and Biological Engineering, 12(6), 143–148. https://doi.org/10.25165/j.ijabe.20191206.4914
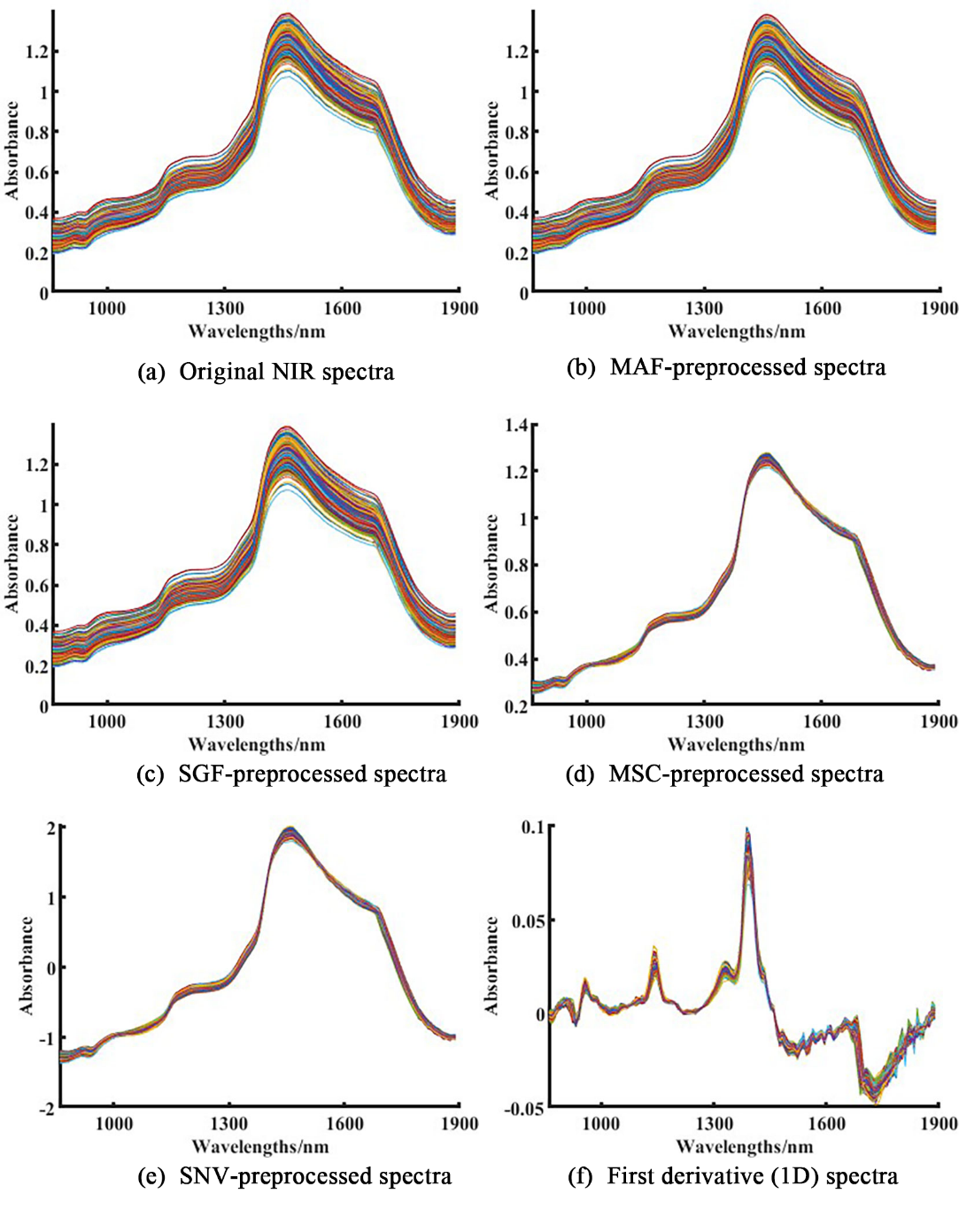

This work is licensed under a Creative Commons Attribution 4.0 International License.
Copyright for this article is retained by the author(s), with first publication rights granted to the journal.
This is an open-access article distributed under the terms and conditions of the Creative Commons Attribution license (http://creativecommons.org/licenses/by/4.0/).