Analysis of Factors Influencing the Price of Beijing Carbon Emission Allowance Based on the VAR Model
Abstract
Effectively constructing a carbon emission trading Market is crucial for China to achieve its goals of carbon peaking and carbon neutrality. However, carbon emission allowance prices are influenced by various factors. This paper employs a Vector Autoregression (VAR) model, using the average transaction price of Beijing Carbon Emission Allowances (BEA) as the research subject. It analyzes related indicators from four aspects: energy prices, macroeconomic conditions, environmental factors, and public sentiment. The results show that among energy price factors, coal and oil prices have a positive impact on BEA prices, while natural gas has a negative impact. Macroeconomic factors initially have a negative impact, followed by fluctuating recovery. Environmental factors, such as the Air Quality Index (AQI) and maximum temperature, initially have a positive impact on BEA prices, followed by fluctuating recovery, while the minimum temperature has the opposite effect. Public sentiment has a significant negative impact on BEA price fluctuations. The empirical results of this study can help investors better understand the changes in the prices of carbon emission allowance, provide references for policymakers, and offer foundations for constructing a national carbon emission trading market that reflects comprehensive and accurate information.
References
Cai, T., Lin, R., & Zhang, X. (2023). A comparative study on the marketization of carbon emission trading in China and Europe—From the perspective of national finance. Journal of Financial Economics Research, 38(2), 127–143.
Deng, G., Zhao, S., Yu, X., Wang, Y., & Li, Y. (2025). An enhanced secondary decomposition model considering energy price for carbon price prediction. Applied Soft Computing, 170. https://doi.org/10.1016/j.asoc.2024.112648
Jiang, M., Che, J., Li, S., Hu, K., & Xu, Y. (2025). Incorporating key features from structured and unstructured data for enhanced carbon trading price forecasting with interpretability analysis. Applied Energy, 382. https://doi.org/10.1016/j.apenergy.2025.125301
Qiao, N., Zhang, C., Zhang, J., et al. (2024). Key influencing factors and prediction research of carbon emission trading price—A comparative analysis based on MIV-LSTM and other models. Price: Theory & Practice, 9, 139–148.
Shen, L., & Luo, M. (2022). Multi-factor prediction research of carbon emission trading price based on LSTM algorithm. Price: Theory & Practice, 7, 64–68.
Sun, Q., Chen, H., Long, R., & Chen, J. (2024). Integrated prediction of carbon price in China based on heterogeneous structural information and wall-value constraints. Energy, 306. https://doi.org/10.1016/j.energy.2024.132483
Wang, Z., Wei, Y., & Wang, S. (2025). Forecasting the carbon price of China's national carbon market: A novel dynamic interval-valued framework. Energy Economics, 141. https://doi.org/10.1016/j.eneco.2024.108107
Xiong, P., & Wang, Y. (2024). Research on the transmission path of China's coal price and carbon emission trading price. Prices Monthly, 2, 11–20.
Xu, Y., & Lien, D. (2025). How do carbon markets interact with energy-intensive sectors? Evidence from price connectedness. International Review of Economics & Finance, 98. https://doi.org/10.1016/j.iref.2025.103857
Zhou, K., & Li, Y. (2019). Influencing factors and fluctuation characteristics of China’s carbon emission trading price. Physica A: Statistical Mechanics and its Applications, 524, 459–474. https://doi.org/10.1016/j.physa.2019.04.249
Zhou, X., Xing, S., Xu, J., Tian, J., Niu, A., & Lin, C. (2025). Impacts of climate change risk and economic policy uncertainty on carbon prices: Configuration analysis from a complex system perspective. Journal of Environmental Management, 373. https://doi.org/10.1016/j.jenvman.2024.123622
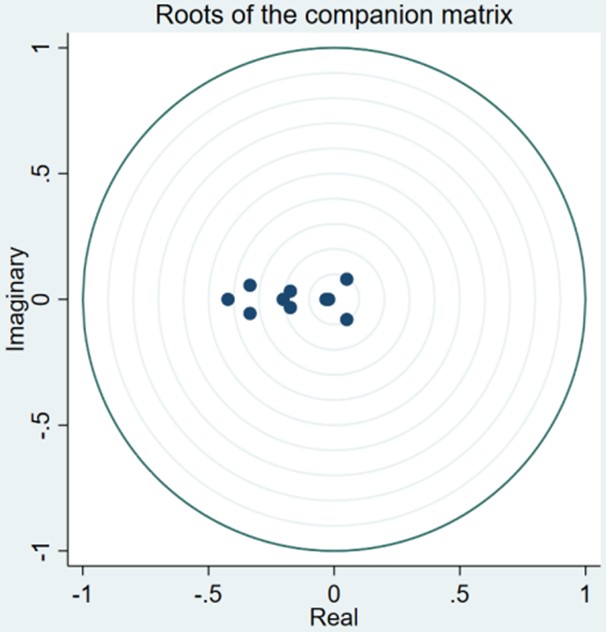

This work is licensed under a Creative Commons Attribution 4.0 International License.
Copyright for this article is retained by the author(s), with first publication rights granted to the journal.
This is an open-access article distributed under the terms and conditions of the Creative Commons Attribution license (http://creativecommons.org/licenses/by/4.0/).