China's Economic Growth from a Comprehensive Analysis Perspective: A Synergistic Application of GRA, PCA, and GRU Models
Abstract
In the context of globalization and economic integration, accurately predicting China's quarterly GDP growth rate is crucial for macroeconomic decision-making. This paper proposes a comprehensive forecasting framework that combines Grey Relational Analysis (GRA), Principal Component Analysis (PCA), and a Gated Recurrent Unit (GRU)-based neural network model to improve prediction accuracy. The study first identifies economic indicators highly correlated with GDP growth through GRA. It then extracts key variability from multidimensional data using PCA, constructing a "China Macroeconomic Vitality Index." Simultaneously, a GRU model, combined with error correction and Bayesian optimization techniques, significantly enhances prediction accuracy. The results demonstrate that the optimized GRU model exhibits significant reductions in evaluation metrics such as SMAPE, MAE, and MSE, indicating good forecasting performance. Additionally, SHAP value analysis reveals the specific impact of each economic indicator on GDP growth, providing a basis for macroeconomic decisions.
References
[2] Sun, W., Yang, J., Yang, Y., et al. (2018). Analysis and prediction of China's GDP based on SARIMA model. China Collective Economy, 2018(36), 78–80.
[3] He, Q. (2018). Exploring the idea of using big data to predict quarterly GDP growth rate. China Statistics, 2018(09), 9–12.
[4] Yi, Y., Huang, D., & Wang, X. (2024). GDP nowcasting based on macro big data. China Economic Quarterly, 24(03), 843–860. https://doi.org/10.13821/j.cnki.ceq.2024.03.10
[5] You, X., Sun, D., & Lin, F. (2010). Application of combined model in China's GDP prediction. Journal of Langfang Normal College (Natural Science Edition), 10(2), 87–89.
[6] Wang, S., Chen, A., Su, J., et al. (2009). Application of combined prediction model in China's GDP prediction. Journal of Shandong University (Natural Science Edition), 44(2), 56–59.
[7] Zhang, J. (2016). Short-term prediction of China's GDP based on time series. Commerce (Natural Science Edition), (15), 204–206.
[8] Li, N., & Xue, J. (2013). Prediction of China's GDP growth based on the optimal ARIMA model. Statistics and Decision, (9), 23–26.
[9] He, Y., Sun, Y., & Gao, Y. (2024). Quarterly GDP prediction based on SARIMA-LSTM model. Times Economy and Trade, 21(10), 28–32. https://doi.org/10.19463/j.cnki.sdjm.2024.10.011
[10] Wu, Y., Luo, J., Zhao, Z., et al. (2024). GDP prediction of Inner Mongolia Autonomous Region based on LSTM neural network. Journal of Inner Mongolia University of Technology (Natural Science Edition), 43(04), 296–301. https://doi.org/10.13785/j.cnki.nmggydxxbzrkxb.2024.04.002
[11] Guo, Q., & He, Y. (2014). GDP prediction model based on DFA method and BP neural network. Statistics and Decision, (08), 82–84. https://doi.org/10.13546/j.cnki.tjyjc.2014.08.030
[12] Xiao, Z., Liu, L., Zhao, T., et al. (2020). Can deep learning neural network improve GDP prediction ability? Research on Economics and Management, 41(07), 3–17.
[13] Liang, L., & Chen, Y. (2023). Quarterly GDP prediction based on deep learning neural network. Statistics and Decision, 39(02), 24–29. https://doi.org/10.13546/j.cnki.tjyjc.2023.02.005
[14] Yu, L. (2017). Application of ARIMA model in China's GDP prediction. Times Finance, (21), 180+185.
[15] Xue, Q., Mou, F., & Tu, Z. (2017). Application of combined prediction methods in Chongqing GDP prediction. Journal of Chongqing Technology and Business University (Natural Science Edition), 34(1), 56–63.
[16] Yuan, Y. (2021). Research on the measurement of China's quarterly GDP growth rate based on the MIDAS model (Doctoral dissertation). Jinan University.
[17] Fu, X. (2022). Research on nowcasting China's quarterly GDP based on dynamic factor model (Doctoral dissertation). Southwestern University of Finance and Economics.
[18] Ding, L. (2010). Time series model and prediction of GDP per capita in Heilongjiang Province. Journal of Harbin Normal University (Natural Science Edition), 26(6), 16–18.
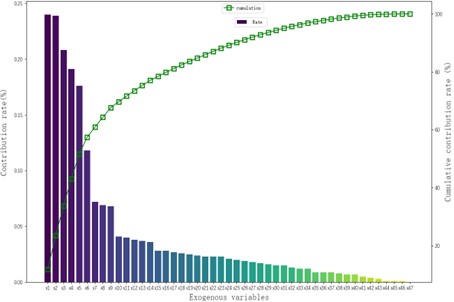

This work is licensed under a Creative Commons Attribution 4.0 International License.
Copyright for this article is retained by the author(s), with first publication rights granted to the journal.
This is an open-access article distributed under the terms and conditions of the Creative Commons Attribution license (http://creativecommons.org/licenses/by/4.0/).